Why we fly: Revolutionizing wheat phenotyping with drones
CIUDAD OBREGÓN, Mexico- Ravi Singh compares plots of wheat lines growing in the fields of Obregón to determine which lines have potential as new varieties. Relying on reams of statistical breeding data and an experienced eye, the head of bread wheat improvement at the International Maize and Wheat Improvement Center (CIMMYT) evaluates plants for resistance to rusts and other diseases, height, tillering ability, grain fill or the mass and size of the spikes, and general vigor or robustness. After detailed evaluation, the fate of the plant is determined: it is selected for advancement and harvested for seed for a yield trial, or it is passed over. With his team, Singh surveys tens of thousands of small wheat plots each season.
In a new program supported in part by the Delivering Genetic Gain in Wheat (DGGW) project and by the USAID Feed the Future Innovation Lab at Kansas State University (KSU) and the US Department of Agriculture’s National Institute of Food and Agriculture (USDA-NIFA), Singh’s analysis may soon be enhanced by a system that predicts the potential of a plant by identifying qualities impossible to see from the ground.
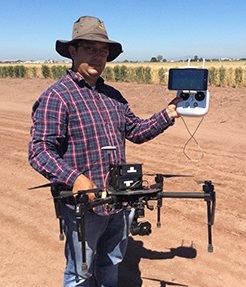
Four years ago, Suchismita Mondal, a plant breeder with CIMMYT, began working on high throughput phenotyping (HTP) with the agronomy group. At the time, HTP meant fitting airplanes with multispectral cameras and flying them about 270 meters above crops. The data captured by those flights helped farmers determine the most efficient use of inputs such as timing and amounts of fertilizer on fields. But, at that height, the cameras could not focus on small (1 sq. meter) plots, and the low photo resolution was only useful for giving general data. Today, in collaboration with KSU scientists led by Jesse Poland, Mondal and her team are working on a better solution for prediction modeling using phenotypic information on large yield trial plots.
Low-flying eyes in the sky
Drones, or unmanned aerial systems (UAVs), can be thought of as programmable, mechanical hummingbirds. Since drones have become less expensive and more controllable for low altitude flying in recent years, they are the ideal tool for Mondal’s experiments with agricultural surveys. At this year’s field day during CIMMYT’s Visitors Week in Obregón, Mondal demonstrated the two drones her team is using to survey small plots and yield trials.
“They can provide more information than an on-the-ground visual inspection can reveal,” Mondal explained. “The drone cameras reveal the effects of disease and stresses on a particular line.”
This is the second year that Mondal’s team is using the drones, which are still in the testing phase. In the first year, they used the drones to collect data for small plots of F5 and F6 segregating lines. This year, when the lines were grown out in yield trials, the drones collected similar data — to validate the predictive models.
More than pretty pictures
The specialized cameras mounted on the drones are the key to their ability to collect specific information. For instance, a multi-spectral camera reads five different spans of the light spectrum to reveal different qualities in the canopy, such as biomass and other indicators of grain yield. A thermal camera measures evaporative cooling of the plot canopy, which lets researchers measure how well a plant utilizes water. A cooler canopy indicates better water use by the plants, and will usually give higher yield. These are important indicators for drought and heat tolerance. Finally, a camera can measure the color of the canopy using a lens that detects red-green-blue RGB wavelengths. Greener leaves indicate efficient nitrogen uptake as well as overall vigor — more indicators of yield.
Richard Brown, a UAV pilot with Poland’s lab at Kansas State University (KSU), was in Obregón to run the technical aspects of the drone program. Using GPS coordinates, he programs the drone with a flight plan to precisely travel back and forth across a plot — much the way a tractor with auto-steering travels across a field. Once in the air, the drones fly on autopilot, moving at about 12 kilometers per hour and staying about 20 to 60 meters above the canopy depending on the camera used and weather conditions.
On the ground, the operator stands by in case the camera stops transmitting to his handheld tablet. Each drone has about 20 minutes of flight time. To continuously fly the drones and cover the scope of the 18 hectares of breeding nurseries, the team constantly rotates 50 batteries between use and being charged.
Before each flight and battery change, the cameras must be calibrated for accurate readings. The drone hovers above a row of panels on the ground ranging from white to nearly black. To the naked eye, they look like a series of gray tiles, but they throw off different spectrums of light that the drones can detect.
From data to predictions
The drones are also being used to gather data on plant growth throughout the season. Researchers can analyze these data to determine the optimum time to take measurements for predictive value. The data from a single season fills a 3 to 4 terabyte hard drive, which Brown uploads to the high performan
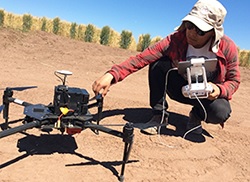
ce computing cluster at KSU. It’s an enormous amount of information to sift through. “The pictures are stitched together to give a map of the area with all the detailed data captured by the camera,” Brown explained. “Using those detailed maps, the HTP team makes the predictive models.”
Currently, the HTP program at CIMMYT is flying two drones: one was purchased with funds from the DGGW project, and the other is provided through the USAID Innovation Lab and USDA-NIFA. Each drone carries one of three cameras. Several more seasons of testing will allow scientists to refine the predictive models and determine the most efficient times and conditions to collect data.
Did the lines that the drone cameras indicated were high yielding actually bear out? Initial results from large size field plots suggest that the data from the drones can indeed be used to predict how well a line will perform. A previous CIMMYT/Cornell/KSU study, led by plant breeder Jessica Rutkoski and the HTP team, showed that these data can improve genomic selection prediction by up to 70 percent. Singh says he can only see visible agronomic traits when selecting, however invisible traits can possibly be selected using the HTP data. Combining both should allow breeders to select better performing wheat lines
For decades, farmers around the world have benefited from the work of experienced wheat breeders like Ravi Singh, and their time- and data-tested ability to pick wheat lines with desirable characteristics. Backed by the data generated by low-flying mechanical birds, and analysis by high throughput phenotyping teams, genomic selection prediction modeling could help make the plant breeders’ job even easier and more successful leading to delivering higher genetic gains.